CHIP is a pioneer in Natural Language Processing (NLP), or the computerized reading of human language datasets in order to read, understand, and derive meaning.
Projects
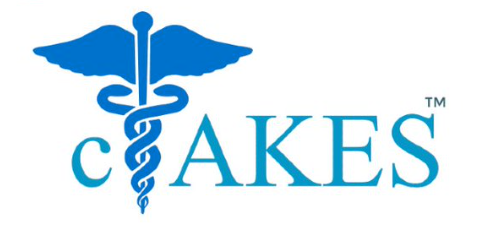
Apache clinical Text Analysis and Knowledge Extraction System (cTAKES) is a widely used, open source and free tool for clinical natural language processing (NLP). Unlike general purpose NLP tools, cTAKES is specialized for clinical texts, incorporating Unified Medical Language System (UMLS) resources for finding medical concepts and packaged with machine learning models trained on gold standard clinical texts. Apache cTAKES has NLP use that extends beyond clinical care. Apache cTAKES became the first and only top-level Apache Software Foundation biomedical informatics software in 2013. In 2019, Apache cTAKES was named one of the 20 most influential Apache projects.
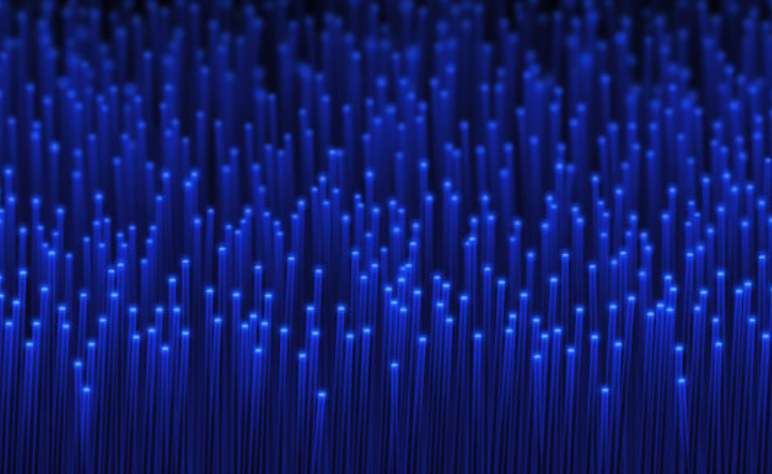
CHIP researchers develop novel methods for information extraction to facilitate automatic/unsupervised/minimally supervised extraction of specific discrete cancer- related data from various types of unstructured electronic medical records. Our two main use cases are cancer deep phenotyping for translational science (DeepPhe) and a platform for cancer surveillance by the cancer registries (DeepPhe*CR).
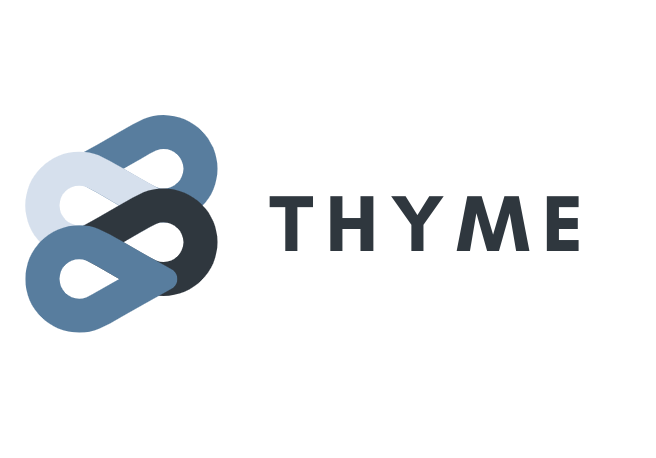
Temporal Histories of Your Medical Event (THYME) uses temporal relations in processing free text. Understanding the timeline of clinically relevant events is key to the next generation of translational research where the importance of generalizing over large amounts of data holds the promise of deciphering biomedical puzzles.
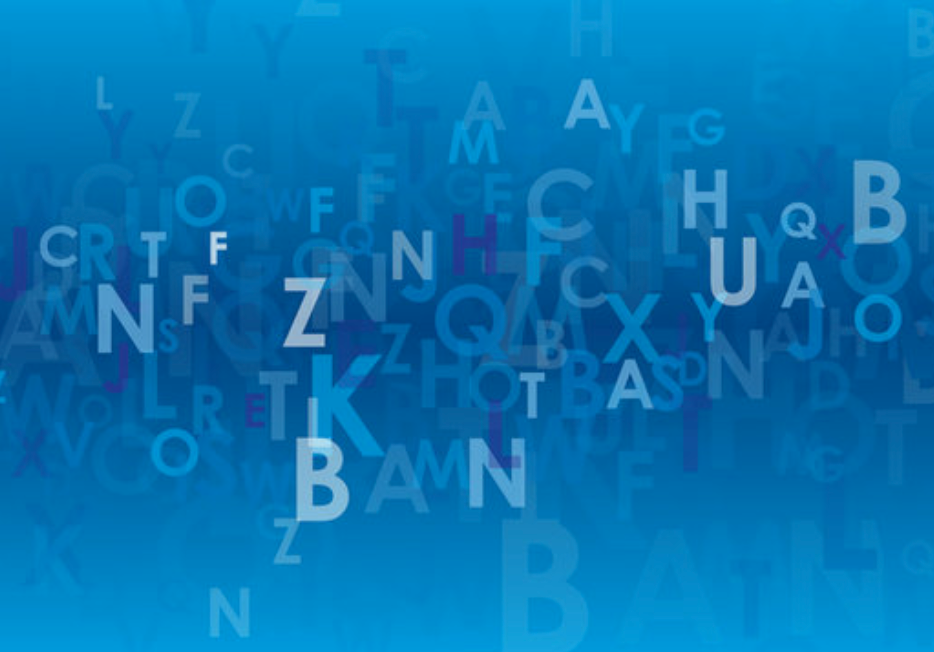
The Health Natural Language Processing (hNLP) Center targets a key challenge to current hNLP research and health-related human language technology development: the lack of health-related language data. The Center’s primary activities are to: provide a repository and a data curation, distribution and management point for health-related language resources, support sponsored research programs and health-related language-based technology evaluations, and engage in collaborations with US and foreign researchers, institutions and data centers.
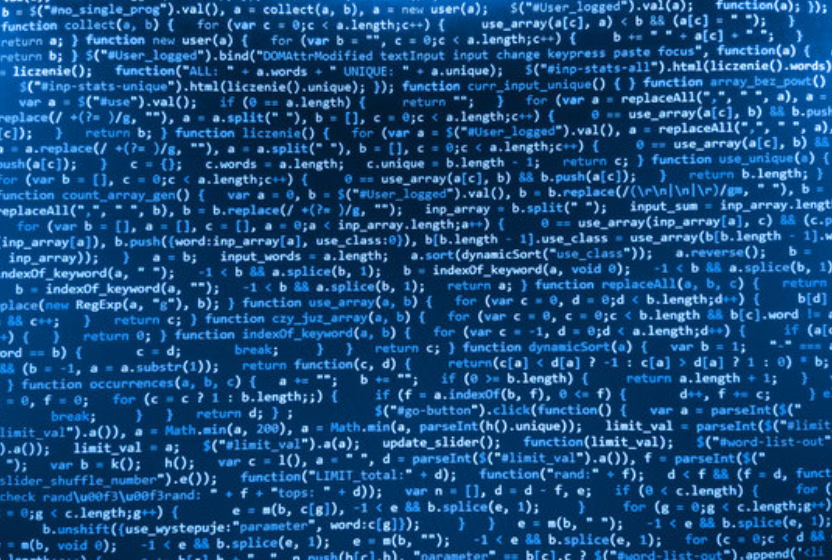
Our goals are to apply the best performing NLP methods to impactful biomedical uses cases to advance the science of biomedicine and clinical care, such as, pediatric pulmonary hypertension, rheumatoid arthritis, inflammatory bowel disease, artery aneurysms, early childhood obesity, autism spectrum disorder, polycystic ovary syndrome, and methotrexate-induced liver toxicity.
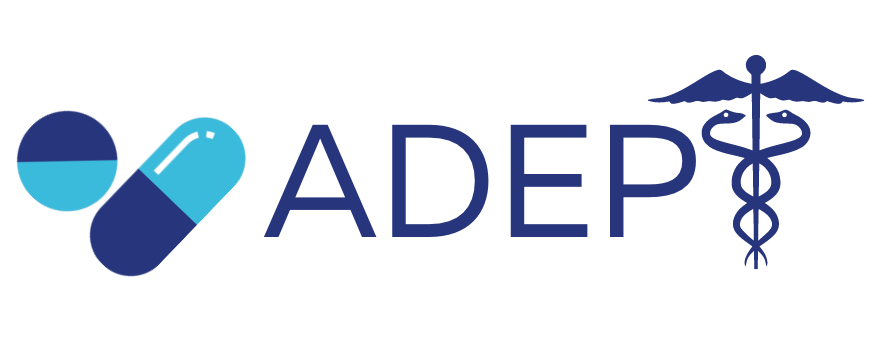
The Adverse Drug Event Presentation and Tracking (ADEPT) system employs an open sources NLP pipeline to identify in clinical notes mentions of medications and signs and symptoms potentially indicative of adverse drug events. ADEPT presents the output to human reviewers by highlighting these drug-event pairs within the context of the clinical note.
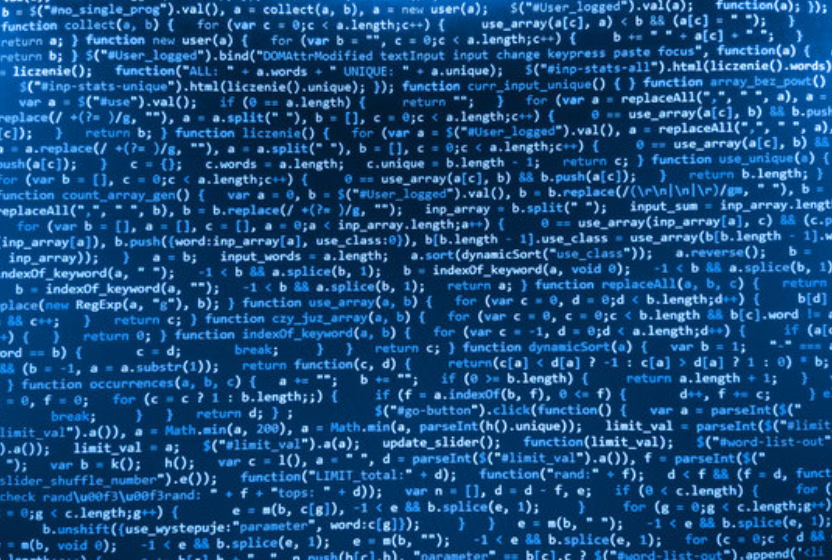
Our goals are to apply the best performing NLP methods to impactful biomedical uses cases to advance the science of biomedicine and clinical care, such as, pediatric pulmonary hypertension, rheumatoid arthritis, inflammatory bowel disease, artery aneurysms, early childhood obesity, autism spectrum disorder, polycystic ovary syndrome, and methotrexate-induced liver toxicity.